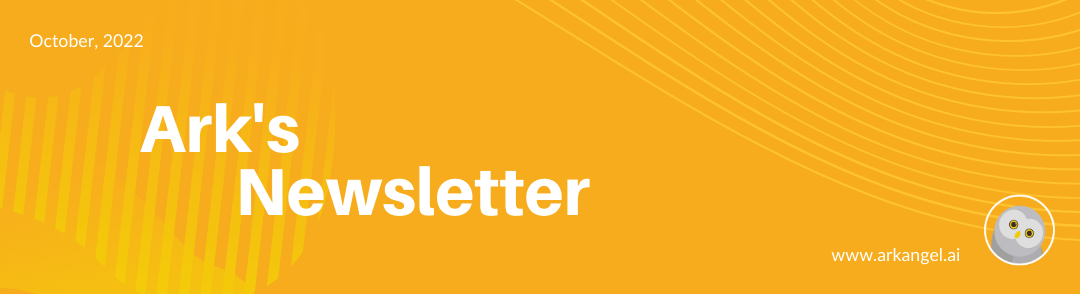
Can AI fight the mental health crisis?
Reading time: 5 minutes, 30 seconds
In the last year, we’ve seen close peers who struggle with mental crises, a fellow entrepreneur who committed suicide, and the story from a friend who works at the mental health clinic who witnessed one student suicide while on the phone due to a service collapse. As it is something close to Arkangel AI, we wanted to cover it in this piece, shedding some light on the challenges ahead and some ideas on how we solve them.
Nearly 1 billion people live with a mental disorder today.
As we build and lead a health tech company, we noticed that we don't talk enough about mental health. I started to learn and found that 2 out of 3 people with a mental disorder go untreated in the United States. Today about 48% of your team has experienced poor mental health at their job. And 57% of people with a mental illness do not receive treatment in a given year.
Gaps in care
Today’s hospitals are challenged because of the increase in mental health crises. A report from the WHO mentioned that the global prevalence of anxiety and depression [increased by a massive 25%](https://www.who.int/news/item/02-03-2022-covid-19-pandemic-triggers-25-increase-in-prevalence-of-anxiety-and-depression-worldwide#:~:text=Wake-up call to all,mental health services and support&text=In the first year of,Health Organization (WHO) today.) in the year after COVID-19. What’s really worrying is that the report shows this crisis is affecting young people the most as they are “[disproportionally at risk of suicidal and self-harming behaviours](https://www.who.int/news/item/02-03-2022-covid-19-pandemic-triggers-25-increase-in-prevalence-of-anxiety-and-depression-worldwide#:~:text=Wake-up call to all,mental health services and support&text=In the first year of,Health Organization (WHO) today.)”. People like us and our friends.
People with mental crises are twice as likely to develop type 2 diabetes and 3 to 5 times more likely to suffer a stroke. Fortunately, we noticed an increase in interest towards the end of 2021 to improve, but today still there is a growing demand for mental health services coupled with a shortage of skilled personnel.
As with any medical condition early detection is key both for cost reduction, and better patient outcomes. There is plenty of evidence that shows how we can predict critical events associated with multiple conditions, that go from hospital readmission to hypertension and diabetes (You can see it here, here, here, and here).
The problem is the manual review of patient records is just impractical for mental healthcare workers due to the sheer volume of data points that need to be considered. Timely treatment can prevent exacerbating the symptoms that lead to such crises and subsequent hospitalization. Additionally, we already know that for every $1 invested in mental health support it returns $4 in improved health and productivity.
A solution
In a recent study published in the Nature Medicine journal, researchers evaluated the effectiveness of machine learning in the prediction of mental health crises.
A team of researchers developed an AI model to predict mental health crises over a period of 28 days in the future. The goal was to estimate the risk of a mental health crisis, optimize healthcare staff allocation and prevent the crisis.
The premise is that we have patterns in the data that can predict future mental health crises in Electronic Medical Records (EHR), despite the noise and errors.
Dataset
The team used a dataset from the Birmingham and Solihull Mental Health NHS Foundation Trust collected over 7 years (2012-2018) which included:
- 17,122 patients aged between 16 and 102 years containing 5,816,586 records.
- The dataset had 60,388 crises with a mean of 24 events per episode.
- They included only patients: Who had at least 2 crisis episodes in their medical records to make them suitable for the training and testing phases.With more than 3 months of records in the EHR to make their historical sufficient for the algorithm to learn from.
The prediction target:

Fig 1 from the paper: Example of a crisis episode timeline: crisis onset is the first crisis event of a crisis episode that follows a stable week (that is, a week without crisis events).
- They label the event as a ‘crisis’ when a patient required one of the following urgent mental health crisis services in a given week: Emergency assessment, inpatient admission, home treatment assessment, and hospitalization.
- The 28-day prediction window was selected due to aligning with the service-oriented approach of the trust.
The top 8 most predictive variables
They performed SHAP value calculations to find the most predictive variables and got these:
- Weeks since the last crisis
- Never hospitalized
- Number of crisis episodes
- Number of years since the first visit
- Weeks since the last missed appointment
- Age
- Not diagnosed
- F6 Disorders of adult personality and behavior
The best algorithm
They trained multiple models and found that:
- XGBoost showed the highest accuracy across different metrics, followed by
- Neural Networks,
- Random Forest,
- Logistic Regression.
Results
They benchmarked the model against two baseline classifiers: a clinical-practice-based baseline model (decision tree) to emulate a doctor’s decisions to assess the risk of relapse, and a diagnosis-based logistic regression that relied on diagnosis and time elapsed since the last crisis.
Model performance (The closer to 1.0 in both cases, the better):
- The area under the receiver operating characteristic (AUROC):The ML model: 0.797 (95% confidence interval (CI) 0.793–0.802),The clinical-practice-based baseline: 0.736 (95% CI 0.733–0.740)The diagnosis-based baseline: 0.746 (95% CI 0.741–0.750).
- An average precision (AP):The ML model: 0.159 (95% CI 0.154–0.165)The clinical-practice-based baseline: 0.092 (95% CI 0.090–0.094)The diagnosis-based baseline: 0.092 (95% CI 0.089–0.094).
Key insights:
- If there was no info about the patient for >1 year the metrics dropped. (AUROC 0.617)
- Patients with at least one record in the previous month showed stable results. (AUROC 0.765)
- A long history of the patient in the EHR improved the prediction performance: Patients who visited for the first time the site in the previous 6-months (AUROC 0.794)Patients whose EHR the first record dated 5 years back (AUROC 0.816)
Clinical practice results:
The researchers tested the algorithm on a 6-month prospective study with multiple sites to see the reactions and results from healthcare professionals and found:
- 7% disagreement (n = 65) of all predictions over 6 months.
- Predictions are clinically valuable in managing caseloads or mitigating the risk of crisis in 64% of cases.
Data Fig. 9 from paper: Examples of features that contribute to the predicted risk score.
Conclusion
Today we have a busy clinical setting, where a manual review of large quantities of data across many patients makes proactive mental health care decisions unusable, unsustainable, and error-prone.
The future will shift the focus from manual review to continuous analysis of data to enable large-scale continuous data review to catch things before it is too late.
We are facing a new type of pandemic. A lot of us will likely feel or experience a mental health crisis at some point in our lives. Yet there are solutions today to help us detect, prevent and help more people fight back against these challenges, and maybe AI could be part of that solution.
Whenever you're ready, there are three other ways we can help you:
1. **Work with us 1:1:** Book a discovery call to bring the power of AI to your firm.
2. The Ark Library: Take a look at the free resources we have to bring AI to your day-to-day.
3. Join our community for short and sweet tips: If you are the type of person who finds it helpful to receive short, daily tips, then follow us on LinkedIn. We post a few short tips each day.
Journal references (And other good reads):
- Garriga, R., Mas, J., Abraha, S. et al. Machine learning model to predict mental health crises from electronic health records. Nat Med 28, 1240–1248 (2022). https://doi.org/10.1038/s41591-022-01811-5 (link)
- Van Le, D., Montgomery, J., Kirkby, K. C. & Scanlan, J. Risk prediction using natural language processing of electronic mental health records in an inpatient forensic psychiatry setting. J. Biomed. Inform. 86, 49–58 (2018).
- Ye, C. et al. Prediction of incident hypertension within the next year: prospective study using statewide electronic health records and machine learning. J. Med. Internet Res. 20, e22 (2018).
- Arcadu, F. et al. Deep learning algorithm predicts diabetic retinopathy progression in individual patients. NPJ Digit. Med. 2, 92 (2019)
- Hyland, S. et al. Early prediction of circulatory failure in the intensive care unit using machine learning. Nat. Med. 26, 364–373 (2020).
- Li, X. et al. A time-phased machine learning model for real-time prediction of sepsis in critical care. Crit. Care Med. 48, e884–e888 (2020).
- He, Z. et al. Early sepsis prediction using ensemble learning with deep features and artificial features extracted from clinical electronic health records. Crit. Care Med. 48, e1337–e1342 (2020).
- Chen, Q. et al. Predicting suicide attempt or suicide death following a visit to psychiatric specialty care: a machine learning study using Swedish national registry data. PLoS Med. 17, e1003416 (2020).
- Su, C. et al. Machine learning for suicide risk prediction in children and adolescents with electronic health records. Transl. Psychiatry 10, 413 (2020).
If you enjoyed this newsletter, don't forget to share it with others and/or give us feedback so that we can improve it. Until next time!
Best regards,
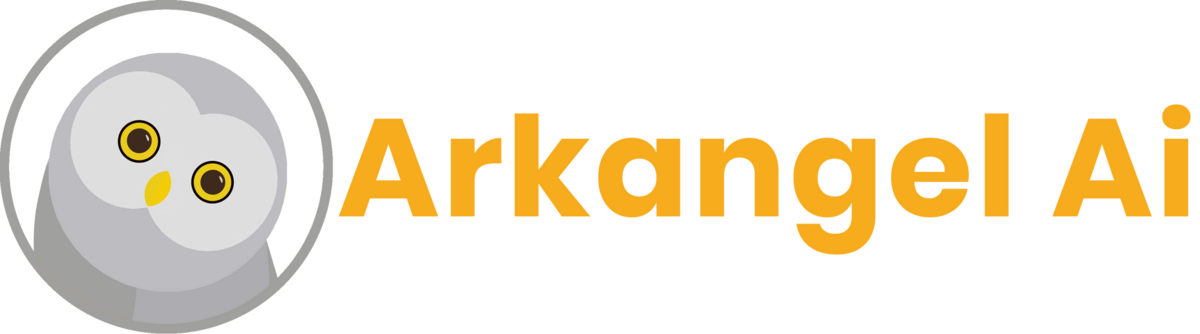